Incorporating Large Language Models into Reading Practices [Resource]
Jonahs Kneitly
What You Will Learn in This Section
In this section, we will examine how generative AI (GenAI) tools may assist with academic reading and research. Examples of content generated by ChatGPT will show how GenAI may be incorporated into a classroom setting. Each section offers suggestions for use and various strategies that could be incorporated for those who wish to allow the use of these tools for assignments. Included throughout are suggestions on how to promote students’ ethical and effective use of these tools and to possibly limit their use if desired. By the end of this section, you should be able to use GenAI to support reading practices.
This chapter is divided in the following sections. Click the links below to jump down to a section that interests you:
- Author Reflection: How has my understanding of AI evolved?
- How can LLMs and other GenAI help with academic reading?
- How might LLMs enrich active reading?
- How can LLMs help with collaborative reading?
- What problems should be avoided?
- What is the point of the caveats placed in AI-generated content?
- If it generates inaccuracies, why use it?
- What strategies can facilitate effective reading?
- What is the best strategy for better information?
- How can educators guide students in using LLMs for academic reading and research?
- What considerations should we share with our students before incorporating LLMs into their reading and research process?
Author Reflection: How has my understanding of GenAI evolved?
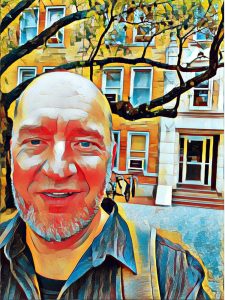
My immediate reaction to including these tools in my classroom was “Never!” After being involved in this project, however, I see that my stance was unconsidered and unachievable. Embracing these tools and integrating them into classrooms as digital literacy tools is the best option to support their ethical and effective use. These GenAI are highly capable tools, but they are not perfect. Having the skills to recognize the weaknesses and leverage the strengths of these tools will help our students build successful careers in a world that is becoming more reliant on this technology.
Jonahs Kneitly (see fig. 1)[1] teaches rhetoric and composition in Texas A&M University’s Department of English, where he is a Ph.D. student studying pain and illness in the late 19th and early 20th centuries.
How can LLMs and other GenAI help with academic reading?
Things to Consider Before Integrating an LLM into the Classroom
While Large Language Models (LLMs) and other GenAI can be valuable tools in the classroom, it is important to be familiar with their associated risks. LLMs are designed to appear intelligent and to have personalities, but instructors should exercise caution when using them within a real-time classroom setting as some have inherent bias and have enacted problematic behaviors during conversations. LLMs and other AI also experience machine drift, which is the degradation of a program over time and the resulting shift in output. Though drift may improve the accuracy and quality of AI-generated content, normally function and output deteriorates over time. Because of this temporal variation, it is essential to remain current on issues with these tools.
Enhancing Reading Comprehension
LLMs and GenAI have the potential to enhance reading comprehension and assist in creating educational resources (see table 1). Though they may be used to design rubrics, these tools should not be used to assess student work.
Table 1
Assisting Teachers in the Classroom
LLMs can help generate:
- focused interactive activities
- assignment prompts
- language translation exercises and provide feedback
- lesson plans
- quiz and test questions
- rubrics
- course syllabi
For the best results, GenAI tools should be bolstered by a user’s critical thinking, independent verification, and strong reading skills. Combining an LLM’s assistance with active reading strategies, can heighten comprehension, creativity, and engagement.
Starting Points and Analyzing Texts
LLMs can assist with locating starting points in the reading and research process by summarizing a text’s main points. Prompting LLMs to assist with identifying specific information, analyzing arguments, and interpreting literary texts can provide a beginning point for research. One strategy is building prompts around the Journalistic Six: who, what, when, where, why, and how. Encourage students to think outside the box when eliciting output with these questions. For example, How may be posited in different ways. Students may state how they would like the content delivered: as a paragraph, as a list, or as a haiku. They may also use How to direct the content toward a specific audience: how is this relevant to my university or cohort? Another strategy for focusing prompts is testing a range of similar prompts to determine which is the most effective (see table 2). GenAI tools incorporate common word usage so they can help users identify ideas being discussed around a particular topic or area.
Table 2
Evolving Prompts for Research
Can you identify articles about alternative energy sources?
What are four types of alternative energy sources?
What is the history of alternative energy in the last 20 years?
What alternative energy is most common in this country?
What alternative energy is most common in this state?
Active engagement in the verification of LLM-generated information may expose students to previously unconsidered ideas and familiarize them with ways to validate information. Refining prompts to focus on or eliminate ideas is a critical part of obtaining good information from an LLM. Assuming that the first attempt at a prompt captures the best or most important points may leave critical information unrevealed.
How might LLMs enrich active reading?
Promoting Reading Strategies
LLMs can assist with active reading by demonstrating different reading strategies, such as annotating, summarizing, and note-taking. ChatGPT can discuss how to perform these skills and provide examples to guide practice.
LLMs can engage in conversations, query for concept understanding, and help users forge connections with the material. LLMs can locate relevant textual elements by finding specific information and words within a text.
Stimulating Critical Thinking
LLMs can aid in analysis by identifying logical fallacies, recognizing biases, and evaluating arguments (see example table. 3). By identifying bias around a topic, these tools can provide insights and perspectives that encourage students to engage in deeper thinking and consider multiple viewpoints.
Table 3
ChatGPT lists common misconceptions and biases concerning vegans and veganism.
LLMs can elicit student responses and provide commentary on their answers. This type of interactive question and answer dialogue can foster self-reflection and help refine understanding and expression of ideas. GenAI tools allow students to move at their own pace and explore personal areas of interest (though accessibility, privacy, and confidentiality should be considered carefully).
Take-Aways Concerning Active Reading and Research
Students can interact with LLMs to enhance active reading skills, comprehension, critical thinking, and engagement with reading material. While these tools can provide guidance and support, they cannot replace human critical thinking skills. Students should record and reflect on their interactions with any GenAI tools in order to build critical engagement.
How can LLMs help with collaborative reading?
Building Collaboration Group Contracts
LLMs can generate group agreements for team members to sign. Agreements will be as vague or nuanced as the students’ prompts. Exercises such as this are great introductions to tailoring output from LLMs. Again, students might consider the journalistic questions: how, when, why, what, where, and who to tailor their agreement. Prompts that are more specific can lead to better output. For example, the Journalistic Six were used to construct this prompt for a collaborative group contract.
Making Reading Recommendations
LLMs can suggest readings based on the groups’ interests or specific topics of study. GenAIs can quickly create a curated list of sources to guide the collaborative reading experience. Any text, book, or AI-generated article suggestions should be verified as GenAI may fabricate information and texts to fulfill a prompt. Sometimes, generated content is correct and verifiable. This ChatGPT curated list of LatinX novels for a group of freshmen students contains real novels with real authors that a student can access. Any text, book, or article suggestions generated by AI tools should be verified as they may fabricate information to fulfill a prompt.
Dividing Readings into Manageable Chunks
LLMs can help determine logical divisions for collaborative reading groups. By breaking down the readings into manageable portions, LLMs can distribute reading load among the group members. ChatGPT suggested this breakdown of J.R.R. Tolkien’s The Fellowship of the Ring which is composed of large sections and shorter chapters in order. However, including specific criteria, such as word count, theme, reading level, or a chapter by chapter rotation would focus the LLM’s breakdown. GenAI tools make student-tailored learning possible within a classroom setting and students should be encouraged to experiment with language to obtain different generated content.
Providing Annotation Ideas
LLMs can provide examples to support engagement with a text. LLMs and other GenAI tools can explain and reverse outline a text. However, reverse outlining may take an inordinate amount of time depending on the section’s length. LLMs usually work better with smaller chunks of text. Each annotation should be critically evaluated as these tools cannot determine importance or interest. However, LLMs can identify recurring words, themes, and ideas within a text. For example, ChatGPT generated an annotation of Ernest Hemingway’s The Old Man and the Sea utilizing key words and concepts.
Organizing Group Notes
After group members have read and summarized their assigned sections, LLMs can assist in combining and structuring the collective notes and annotations. These tools can help consolidate the information, categorize key points, and create a cohesive overview of the readings. If students are having trouble getting started, ChatGPT can offer examples of what team-member notes may look like.
Supporting Research Project Presentations
LLMs can help organize research findings, suggest frameworks for the project’s outline, and provide editing assistance for the final output. Assessing the creativity of a group’s presentation may reduce reliance on AI-generated content as human creativity may find methods and modes that are beyond these tools. However, for many of us getting started is sometimes a hard first step. ChatGPT can suggest a presentation framework such as this one for Ernest Hemingway’s The Old Man and the Sea.
What problems should be avoided?
GenAI Doesn’t Think
GenAI tools are not conscious. Though they compose convincing conversations, they lack emotional intelligence, creativity, independent thought, beliefs, or opinions. LLMs and other GenAI cannot assess truth or value and may propagate bias and false information. Problem-solving, critical thinking, identifying crucial or missing information, determining interest, and self-reflection are beyond their capabilities. LLMs and other GenAI cannot discern intent. Classroom discussion and instruction on these tools should stress clarity as an important consideration when refining prompts.
GenAI is not Omniscient
The temporal and informational limitations of each LLM should be explored, considered, and evaluated before and during use. Many LLMs have access to limited libraries. Though utilizing LLMs can jumpstart research, GenAI tools are not search engines. These tools create output based on common usage and this output may not be factual. Verifying all generated information is crucial. See the What strategies can facilitate effective reading? section below for explanation on how to use our modified stasis theory to expedite the reading process.
Different LLMs have different capabilities, including varying abilities to work with visuals and PDFs. New tools are being added and old tools are being updated constantly. Many websites list different AI, LLMs, and GenAI. This list of various AIs is valid as of September 2023, but you may find other lists by exploring the Web or with a Google search.
GenAI Cannot Attribute Content to a Specific Source
Attribution of AI-generated content can be difficult as a determination of exact sources and original authorship is problematic or impossible. However, MLA and APA have issued current guidelines for citing these tools and users should be familiar with them. Privacy and confidentiality should always be considered with these tools as information, including prompts, are permanently linked to individual user names. This type of personalization poses risks such as student privacy, biased information, and development of unhealthy habits.
What is the point of the caveats placed in AI-generated content?
Stating the Truth Up-Front
An LLM’s generated content will sometimes include caveats (see table 4). The following ChatGPT caveats warn users of possible issues including a limited data library on a particular subject. LLM related pedagogy should stress analyzing any caveats to highlight the LLM’s self-recognized limitations.
Table 4
ChatGPT Generated Caveats (emphasis added to highlight important content)
“I apologize for the misunderstanding. In the context of academic reading, there are several approaches that can be used to handle the reading process collaboratively. Here’s a general outline of how it could work.”
“As an AI language model, I don’t have personal abilities or preferences like an individual. However, I can provide general guidance on the different tasks involved in collaborative reading and offer assistance based on my knowledge and capabilities. Here’s a breakdown of each task and how I can assist:”
“These arguments provide a starting point for your article, but it’s important to conduct further research to gather up-to-date information and a comprehensive understanding of the ongoing debates within each topic.”
Incorporating these caveats into discussions will help students develop a critical mindset about information reliability, the limitations of particular tools, whether LLMs or other forms of AI, and the need to supplement LLM-generated content with further research and analysis.
If it generates inaccuracies, why use it?
Easy and Fast vs. Slow and Responsible
The ability to copy/paste makes these tools easy to use. The ability to paste text into an LLM makes it challenging to stop use of GenAI tools. AI-generated content taken without evaluation and verification can seem like a shortcut. Students list running out of time as the most common cause of academic dishonesty. Making efficient time management part of the reading and discovery process may lessen the reliance on LLMs and other AI tools.
Emphasizing individual-created evaluation criteria and time efficient techniques may help curtail time management problems. Critically evaluating and engaging with LLM-generated texts can be a large investment in time. Sometimes students may opt to do the composition work themselves rather than investing the time to verify each fact that is presented within GenAI content. Stressing the time commitment and oversight necessary to engage ethically and effectively with these tools may lessen the appeal for some students. Emphasis on slow and responsible learning should begin with updating your course syllabus to incorporate this type of process.
What strategies can facilitate effective reading?
Stasis Theory as Reading Strategy
We recommend using a modified version of stasis theory to engage with LLM output. Stasis theory incorporates recursive steps centered around four components—conjecture, definition, quality, and policy. These steps can be dove-tailed into prompts to encourage critical engagement. By incorporating this modified stasis theory, students develop a scaffolded approach to evaluating texts. Process, rather than product, becomes critical to understanding and learning.
Stasis theory may be modified and applied in the following manner for active reading:
Conjecture
Conjecture focuses on facts, evidence, and claims. Students should evaluate the credibility of data and examples provided by any LLM. Fact-checking specific claims and seeking additional sources encourages critical thinking and research skills. LLM output, no matter how false or problematic, will appear knowledgeable and complete. Students should be encouraged to look for patterns of inconsistency throughout the text. If a user confronts an LLM, such as ChatGPT, that has provided false information, normally, it will acknowledge its shortcomings. However, it may subsequently create further misinformation to fulfill the prompt.
Definition
Definition calls on the reader to pay attention to key terms or concepts. Students should identify how terms are used, defined, or if they are defined at all. Students should mine any LLM-generated output for ambiguities and alternative interpretations. Critical analysis of generated texts promotes a deeper understanding and allows students to identify the texts’ weaknesses and strengths. Having students argue against an LLM or edit LLM output encourages critical mindset for identifying missing or fallacious information.
Quality
When analyzing the quality of a work, LLMs can help assess the strength of an argument. These tools can help identify logical fallacies, inconsistencies, or unsupported assumptions. They can generate examples of logical tropes and fallacies to aid with understanding. GenAI tools can argue different sides of an issue so have students compare and contrast different viewpoints to gain a well-rounded perspective. Students can engage with texts and the GenAI output to identify different criteria, missing viewpoints, or alternative understandings. This type of critical engagement pushes students beyond immediate acceptance of LLM-generated content which might be shallow or rudimentary.
Policy/Solutions
When examining policy or recommendations, students should evaluate the feasibility, effectiveness, and potential consequences of any action. Encourage students to identify and research alternative perspectives and assess their merits against those presented within GenAI produced content. Critical analysis of implications engages a student’s creative process as part of their reading strategy.
What is the best strategy for better information?
Crafting Effective Prompts
Taking the time to craft effective prompts gives a great return on a student’s invested time and effort. Students should be coached in creating effective research questions which then can be incorporated in prompts using the basic journalistic questions mentioned earlier. A small change in verbiage can greatly affect GenAI output texts. Encourage students to create their own templates based on the Journalistic Six or some other question matrix. Also, across LLMs and at different times, the same question may elicit different responses. Spending time refining verbiage will help students clarify their own thinking about a subject and may lessen the amount of generated misinformation (see table 5).
Table 5
ChatGPT generated content defining Stasis Theory in a 50 and 75 word paragraph which highlights the content’s evolution as word count changes.
Stasis theory is a rhetorical concept used to analyze and categorize the key points of argumentation within a discourse.
In a 50-word paragraph: it involves identifying four stages: conjecture (defining the issue), definition (clarifying key terms), quality (evaluating the facts), and policy (proposing a course of action).
In a 75-word paragraph: stasis theory serves as a systematic framework for dissecting and understanding arguments. It begins with conjecture, where the issue at hand is framed and understood. Next, definition hones in on key terms and concepts, ensuring clarity and shared understanding. Quality scrutinizes the veracity and relevance of facts and evidence presented. Finally, policy addresses the proposed solutions or actions to be taken in response to the identified issue. It helps both rhetoricians and audiences engage more critically with arguments.
How can educators guide students in using LLMs for academic reading and research?
Approach Interactions as a Starting Point
LLMs can be valuable tools to assist with learning. These tools are here to stay, and their use is quickly expanding into different venues. Instructors can spend valuable teaching moments policing these un-policeable tools, or they can create engagement processes for students. This section included techniques and tips that may minimize reliance on these tools. Instructors should consider incorporating GenAI within their personal teaching philosophies. Each instructor should consider whether their courses will prepare students to engage with these tools ethically and effectively.
What considerations should we share with our students before incorporating LLMs into their reading and research process?
Educators can use the language in table 6 to share an abbreviated version of this section with students.
Table 6
Consideration of LLM Use in the Reading and Research Process to Share with Students
AI-generated responses may contain ideas or thoughts that belong to someone as their intellectual property. Some responses may be completely false. Before asking an LLM, such as ChatGPT, or another GenAI to help with reading or research tasks, ask yourself these questions: How would I feel if my paper was culled by an LLM and given to another student with no effort on their part? How would I feel about distributing misinformation that was supplied to me by this tool?
Before using GenAI tools to assist with your reading and research, engage in dialogues with your instructors to determine how you may and may not use these tools in their classes. Ask your instructors what their policies are, what they consider plagiarism, and what type of LLM content they will accept. You should also check the syllabus for university and course policies concerning use of any GenAI.
While LLMs can guide your discovery while reading and researching, they cannot think for you. At this moment, they can’t think at all. They may even fabricate information that incurs real world consequences for you in your coursework.
The purpose of education is to help develop your critical thinking, problem solving and real-life success skills. We encourage you to use GenAI tools to assist your unmatchable human creativity in ways allowed within your courses and institutions.
Attribution:
Kneitly, Johnas. “Incorporating Large Language Models into Reading Practices [Resource].” Strategies, Skills and Models for Student Success in Writing and Reading Comprehension. College Station: Texas A&M University, 2024. This work is licensed with a Creative Commons Attribution 4.0 International License (CC BY 4.0).
Images and screenshots are subject to their respective terms and conditions.
- Jonahs Kneitly, "Image of the author enhanced with Picsart," 2023. ↵